AI Driven Loss Bucketing In Solar Projects
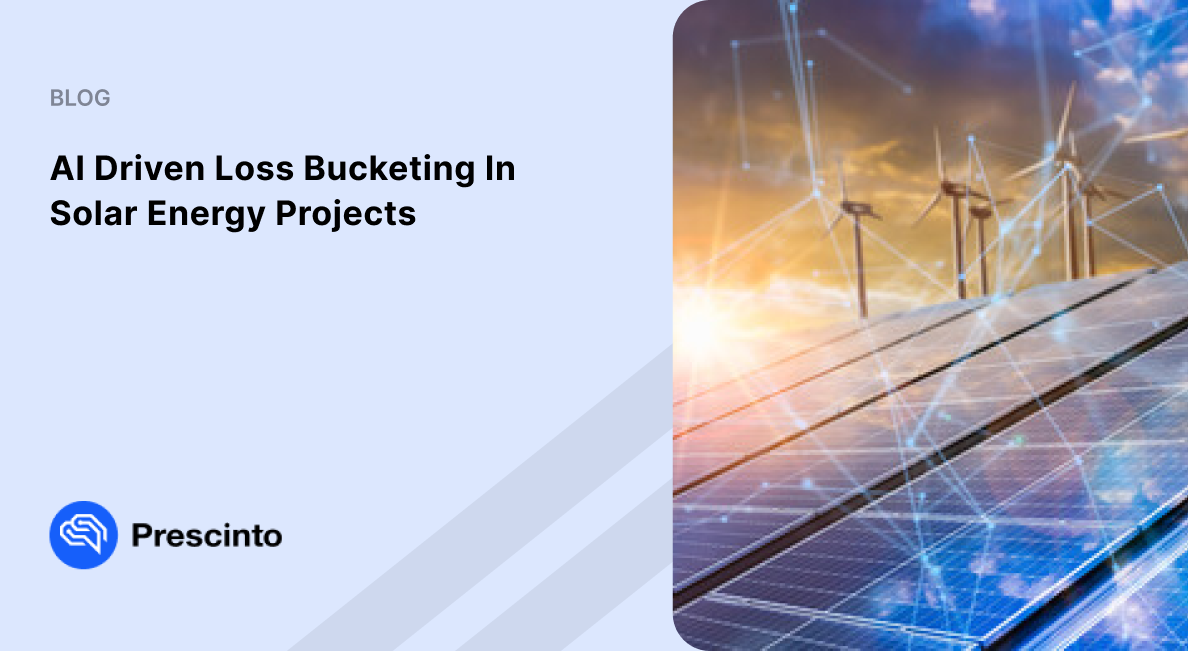
- February 2, 2024
- Prescinto
AI Driven Loss Bucketing in Solar Energy Projects
Solar energy projects are becoming an increasingly attractive option as the world looks for alternative sources of energy. However, solar energy projects come with a set of challenges, which includes managing energy losses associated with these projects. AI driven loss bucketing is a strategy that can help manage these losses more effectively and efficiently, resulting in improved power generation and reduced operating costs.
What is Loss Bucketing In Solar Plant?
Loss bucketing in solar refers to the process of categorizing and quantifying various types of losses that can occur in a solar power generation system. These losses can majorly impact the solar power plant’s overall energy output and efficiency. Loss bucketing involves breaking down the energy losses into specific “buckets,” each representing a different type of loss or inefficiency.
By identifying, quantifying, and categorizing these losses, solar project operators and managers can take corrective actions to optimize energy generation and maximize profits. Operators can combat losses by using AI driven loss bucketing.
Types of Losses in Solar Plants
For solar energy projects, various types of losses can occur, each affecting the overall energy generation differently:
-
Downtime Loss :
The time when a solar plant is not operational due to maintenance, equipment failures, or other issues.
-
Inverter Efficiency Loss:
Reduction in energy output caused by inefficient inverters that convert direct current (DC) into alternating current (AC).
-
AC Loss :
The amount of energy lost during the transmission and distribution of electricity from the solar plant to end-users.
-
Tracker Loss :
Efficiency loss due to tracking systems not aligning the solar panels optimally with the sun’s position.
-
Extreme Weather Loss :
Losses incurred during adverse weather conditions like heavy storms, hail, or hurricanes.
-
Shadow Loss :
Reduced energy output due to shadows cast by obstructions such as nearby buildings or vegetation.
-
Clipping Loss :
Occurs when the solar panels produce power that is more than the inverter’s maximum capacity so that the additional power generated is clipped by the inverter.
-
Curtailment Loss :
Happens when inverters are operated at a capacity that is 95% lower than the maximum capacity. This is intentionally done in cases where the demand for electricity is less.
-
Systemic and Cleaning Loss :
Decreased efficiency due to inefficient device components. For example, an inverter that performs at a maximum AC conversion efficiency of 95% with respect to its rated efficiency of 98.5% is identified as a component facing systemic loss.
-
Snow Loss :
Energy loss during snow-covered periods when solar panels are not generating power.
-
Grid Out Loss :
Energy that is not generated during grid outages.
-
Temperature Loss :
Efficiency loss due to high temperatures, which can reduce solar panel performance. Solar panels have rated power at standard temperature condition (STC), i.e., 25° Celsius. Since solar modules operate at temperatures usually more than the STC in the field, this accounts for some loss in power, which depends on the temperature coefficient of the module.
-
Radiation Loss:
Losses caused by deviations in solar radiation from what was expected.
-
Unidentified Loss (Loss Adjustment):
The discrepancy between actual power generation with losses and the target power generation, often caused by inaccuracies or adjustments in calculations.
Manual Loss Bucketing vs. AI-Driven Loss Bucketing
Traditionally, the process of loss bucketing in solar energy projects has been a laborious and time-consuming task. Solar project operators and managers had to rely on manual data collection, analysis, and categorization to identify the various types of losses affecting energy generation. This process was not only prone to human errors but also highly inefficient, as it required significant time and resources.
Enter Tahoe, Prescinto’s cutting-edge AI-based loss bucketing tool. Tahoe has revolutionized the way solar energy loss bucketing is conducted, bringing automation and precision to a previously manual and inefficient process.
Automating Loss Identification
Tahoe employs advanced artificial intelligence and predictive analytics to automatically identify and categorize different types of energy losses. By analyzing vast amounts of data from solar panels, inverters, sensors, and other sources, Tahoe can quickly pinpoint inefficiencies and areas of improvement.
Customized Analysis
One of the standout features of Tahoe is its ability to tailor the analysis of losses to the specific needs of solar energy projects. Whether it’s tracking downtime, inverter inefficiencies, extreme weather impacts, or any other loss category, Tahoe adapts to the unique requirements of each project.
Top 5 Benefits of AI-Driven Loss Bucketing in Solar energy
The adoption of AI-driven loss bucketing with Tahoe offers a multitude of benefits for solar energy projects:
Improved Efficiency
By automating the loss bucketing process, Tahoe significantly reduces the time and effort required for analysis. Solar project operators can quickly access accurate insights into energy losses, allowing for more proactive decision-making. Tahoe currently identifies 14 losses and accurately categorizes them into the right buckets. These losses include downtime loss, inverter efficiency loss, AC loss, tracker loss, extreme weather loss, shadow loss, clipping loss, curtailment loss, systemic and cleaning loss, snow loss, grid out loss, radiation loss, and temperature loss.
Enhanced Accuracy
Human errors in manual loss bucketing can lead to inaccuracies in identifying and quantifying losses. Tahoe’s AI algorithms provide precise, data-backed assessments, ensuring reliable results. For instance, Tahoe’s pattern recognition algorithm recognizes the events corresponding to shadows, and the losses are calculated by comparing the actual generation of the inverter and its digital twin. This is partially recoverable depending upon the cause of shadows.
Timely Preventative Maintenance
Losses such as cleaning loss in solar plants occur when foreign materials, such as dust, dirt, or bird droppings, accumulate on solar panels, hindering sunlight absorption and reducing photovoltaic cell efficiency. Driven by artificial intelligence, Tahoe, through daily performance analysis, identifies cleaning loss by comparing inverter performance to its best day, categorizing it as a “variable” loss. With Tahoe’s real-time analysis, solar project managers can proactively schedule maintenance and repairs to address identified losses promptly. This reduces downtime and maximizes energy generation.
Cost Savings
The efficiency and accuracy of AI-driven loss bucketing translate into cost savings. Solar projects can optimize maintenance schedules, reduce operational costs, and extend the life of assets, all contributing to improved profitability. By identifying and addressing various types of losses, such as soiling or clipping losses, Tahoe’s AI-driven loss bucketing helps maximize energy generation. This additional energy production contributes directly to increased revenue. For instance, if cleaning losses due to soiling are detected and mitigated promptly, the panels operate at peak efficiency, generating more electricity.
Long-Term Performance
Tahoe’s continuous monitoring capabilities in solar projects play a crucial role in ensuring long-term performance and consistent energy production. By utilizing AI-driven analytics, it detects anomalies and deviations, predicts maintenance needs, and analyzes performance trends to prevent gradual efficiency declines. It also optimizes cleaning schedules based on historical data, advises on equipment upgrades, and minimizes aging-related losses. Tahoe’s data-driven approach enables solar project managers to make informed decisions, safeguarding against potential long-term inefficiencies and ensuring a stable return on investment.
Bottom Line of Loss bucketing
Accurate loss bucketing has the potential to radically transform the solar energy industry. By allowing solar energy projects to accurately identify losses and take corrective action before it’s too late, they can reduce their risk exposure and create more profitable investments. AI-driven tools such as Tahoe (Prescinto’s loss bucketing tool) are revolutionizing the way the renewable energy industry approaches solar energy projects by providing accurate insights into potential losses earlier on in the project’s lifetime. By utilizing AI-driven analytics, it detects anomalies and deviations, predicts maintenance needs, and analyzes performance trends to prevent gradual efficiency declines. Tahoe’s data-driven actionable recommendations enable solar project managers and operators to make informed decisions, safeguarding against potential long-term inefficiencies and ensuring a stable return on investment. Thus, loss bucketing, once an elusive aspect of risk management, has now become a powerful asset for mitigating risks and boosting profitability in renewable energy investments.
- Topics: Loss bucketing solution, Solar
About Author